
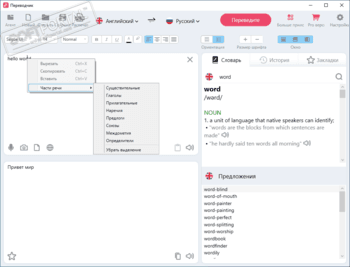
This task is the same as Language Modeling, except that the already-generated words condition each following word. In addition to that, Machine Translation system is trained on a task of a conditional generation. Multilingual models are trained in the same way, with input and output vocabulary covering all input languages over the time, the model learns to identify the language of the input itself, which is often easier than to guess the correct word into the context. This is why only a large amount of tidy texts is needed for (pre-)training the language model (and a few months of computation time), without any costly collection of training samples by a human force.

When training a model for Masked Language Modeling, the weights of the model are iteratively adjusted so that the probability of generating the expected masked word in a given context is maximized. For those familiar with machine learning, I will be a bit more specific: The models learn to understand the text without any explicit training samples, on a task called Masked Language Modeling: here, the model is asked to correctly predict the best-fitting word in a given context. Neural language models that are widely utilised nowadays by all the major translation services are first trained for a general language understanding of huge data sets of text, also referred to as corpus. Better language generation quality can also be achieved with more complicated training objectives, such as de-noising, where the model is trained to reorder the random sequence of words to an original sequence (BART). Image 1: language models are pre-trained for a general understanding of the language, via relatively simple objective, such as Masked Language Modeling, where the model is trained to predict the next word in the sequence.

So what difference can it make, quality-wise? It’s also quite likely that some services use proprietary data sources that, for example, Google surely has available from their web crawls or other services. The selection of the training data, just as the neural model architecture, that each of the services uses, is kept a secret by their providers. Note that although this topic is super interesting from a Data Science perspective, if you came for the comparison of translation services, you don't need to bother about these technicalities. Modern machine translation: how do we translate in 2021īefore picking the right examples for comparison, let’s take a look at what kind of data modern translation systems use to model the translation from one language to another. We’ll acknowledge both the benefits and drawbacks of keeping the translation engine in your hands, from the production perspective.
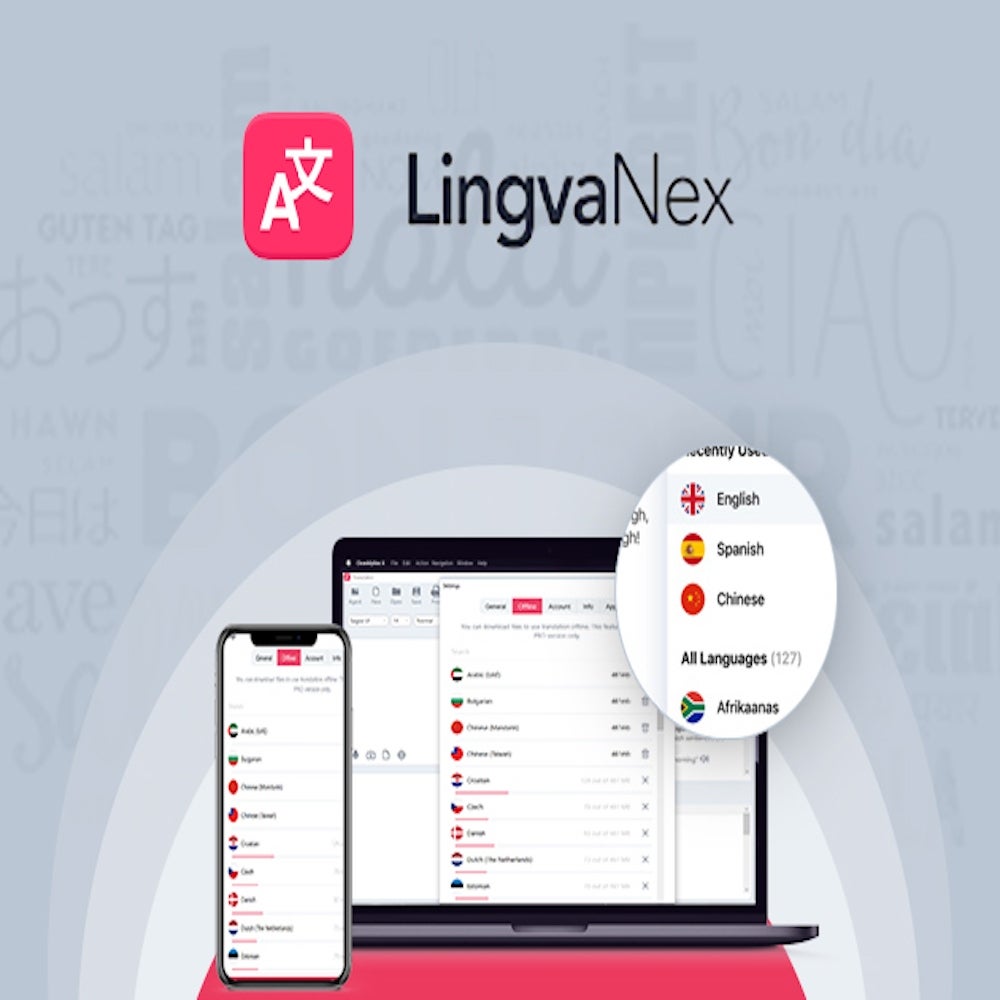
We compare these translation engines to the freely-available options. Additionally, we compare these translation engines to the freely-available options: we’ll outline what you'll need to create a translation engine yourself to give you a glitch of what is possible if you decide to create a useful translation engine yourself. Particularly, we’ll take a look at Google Translate, Amazon Translator and Microsoft Translator. In this article, I’ll share our experience in an overview of commercially-available translation services that you could possibly use in your app or service. Providers of paid translation API vary in both quality and price. However, one quickly finds that there are actually many big corporate providers of translation APIs that support more than a hundred languages. Hence it is also a first choice that comes to my mind when looking for a service to use in a larger-scale application.
LINGVANEX API PROFESSIONAL
When it comes to translation, Google Translate became a tool of everyday professional use perhaps for most of the people.
